The block with personalized recommendations on the website is needed to offer customers other products that may interest them and increase the average check. This is one of the best ways to permanently boost the percentage of sales while simultaneously improving the service. How to do it? The simplest solution is to show the user what they like. These can be things they explicitly expressed interest in—selected or saved to favorites. A slightly more complex solution is to display items that implicitly interest the buyer, such as other products from viewed categories. Additionally, one can showcase items liked by other users and those that are advantageous to sell right now, taking into account the main parameter—the potential buyer’s interest in the product.
But how does a business achieve personalization? Through software algorithms. These are complex mathematical calculations of simple and logical relationships. All that is needed to build them is existing information about products and customers.
In this blog post, we will explore the power of personalization and how to use data to enhance your marketing efforts.
- What is personalization?
- Data Collection;
- Data analysis and utilization;
- Customer segmentation;
- About brands;
- Conclusion.
What is personalization?
Personalization is adapting marketing messages, product recommendations, and other experiences for individual customers based on their unique characteristics and behavior. Examples of personalization include personalized emails, product recommendations, and website content.
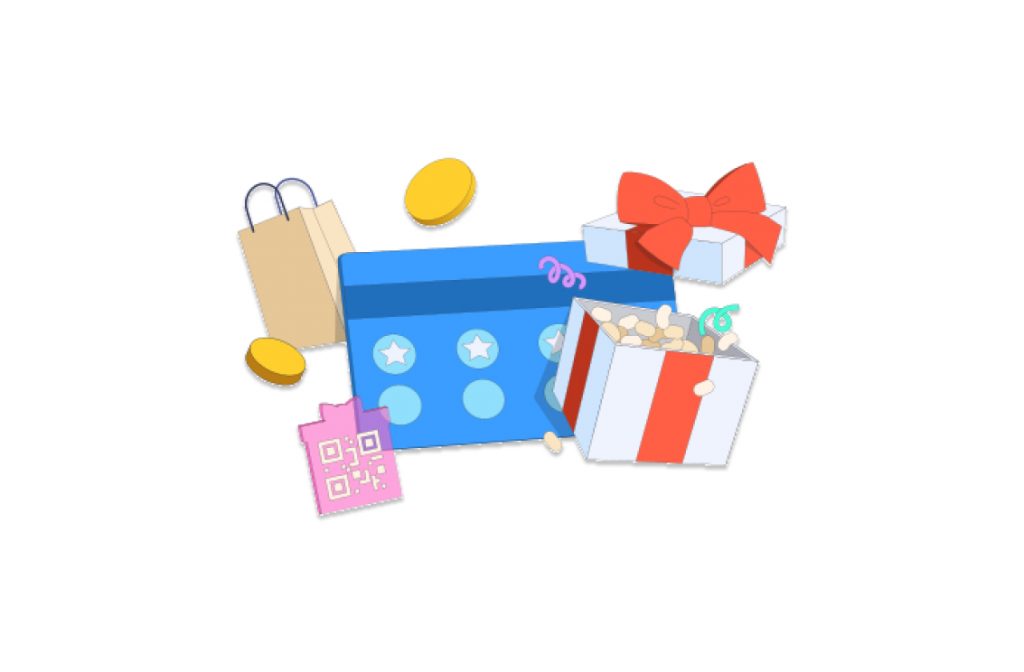
To obtain data from personalized recommendations, the following processing algorithms are used:
Data collection.
To personalize, companies need customer data. Companies can collect various data types, including demographic, behavioral, and transaction data. Data can be classified into two main types: demographic data, which includes age, gender, and location, and behavioral data, which includes clicks, feedback, and website interactions. Transaction data has past purchases, order history, and customer preferences. Data collection methods may consist of surveys, cookies, and CRM software.
The next step is data analysis and utilization.
After companies collect data, they analyze it to identify patterns and insights. This can be done using data analysis and machine learning tools and methods. Personalization techniques and tools that can be used include email segmentation. Product recommendations, and retargeting. For example, companies can use email segmentation to send personalized emails based on previous purchases. Or customer online behavior. They can also use product recommendations to suggest products that match the customer’s interests or purchase history.
Another step is Customer Segmentation.
Customer segmentation is a strategic approach that divides the audience into groups or segments based on common characteristics. Based on the analysis of data, criteria are defined for separating customers. This could include geographic location, demographic characteristics, psychographic, or behavioral factors.
After defining the segmentation criteria, the dividing process of dividing customers into groups or segments based on the chosen methodology begins. Detailed profiles are created for each component, including key characteristics, needs, and preferences. The results are used to adapt marketing strategies and provide personalized services or products for each segment.

All information collected in advance is used to create long-term recommendations. It works like this: once a day, best of all at night, when the servers are less loaded, your recommendation model lists all the parameters and stores the new values in their allotted place in the storage. They will be pulled up to the site when needed, and you can also get them from there for other purposes, such as duplicate personalized emails.
Even basic personal recommendations will already bring you tangible benefits. However, the algorithm can be trained further. For example, use categorization – to divide users and products into groups according to characteristics that are not obvious to artificial intelligence. The algorithm can easily teach who to show cheaper and more expensive goods, as well as allocate those who want to buy in bulk or hunters for discounts.
For optimal outcomes, all these principles need to be combined and adapted to the specifics of your project. Mathematical models are created, machine learning is used, and numerous tests are conducted.
About brands

Many well-known brands successfully use personalization to improve customer relations and increase the effectiveness of marketing strategies. Here are some examples:
- Amazon uses machine learning algorithms to provide personalized product recommendations based on purchase history and views.
- Netflix analyzes the viewing history of each user and uses this data for movie and series recommendations.
- Spotify provides personalized playlists and recommendations based on musical preferences and listening history.
- Google uses user data and their previous queries to provide more accurate and personalized search results.
- Starbucks uses its loyalty program to track purchases and offer personalized discounts.
- Airbnb analyzes users’ travel history and preferences to recommend trips and accommodations.
These examples illustrate how different brands use data to create a more individualized customer experience.
In conclusion, personalized recommendations are a kind of magic—mathematical and statistical magic. Many tests will be required to make a basic algorithm optimal for your project. However, the result will be a tool that boosts sales without requiring intervention and additional investments. Customers often respond positively to the presence of such a block and willingly order products shown in it.
GET IN TOUCH